Publication Summary
Cheminformatics tools for analyzing and designing optimized small molecule libraries
Nienke Moret 1,2, Nicholas A. Clark 3, Marc Hafner 1,2,†, Yuan Wang 4, Eugen Lounkine 4, Mario Medvedovic 3, Jinhua Wang 5,6, Nathanael Gray 5,6, Jeremy Jenkins4 and Peter K Sorger 1,2
1 HMS LINCS and Druggable Genome Centers, Laboratory of Systems Pharmacology, Harvard Program in Therapeutic Science, Harvard Medical School, Boston, Massachusetts 02115, USA.
2 Department of Systems Biology, Harvard Medical School, Boston, Massachusetts 02115, USA.
3 Division of Biostatistics and Bioinformatics, Department of Environmental Health, University of Cincinnati, Cincinnati, OH 45221, USA.
4 Novartis Institutes for BioMedical Research Inc., 181 Massachusetts Avenue, Cambridge, MA 02139, USA.
5 Department of Cancer Biology, Dana-Farber Cancer Institute, Boston, MA 02115, USA.
6 Department of Biological Chemistry and Molecular Pharmacology, Harvard Medical School, 360 Longwood Avenue, Longwood Center 2209, Boston, MA 02115, USA.
† Present address: Department of Bioinformatics and Computational Biology, Genentech Inc., 1 DNA Way, South San Francisco, California 94080, USA.
Cell Chemical Biology 26, 1–13
https://doi.org/10.1016/j.chembiol.2019.02.018
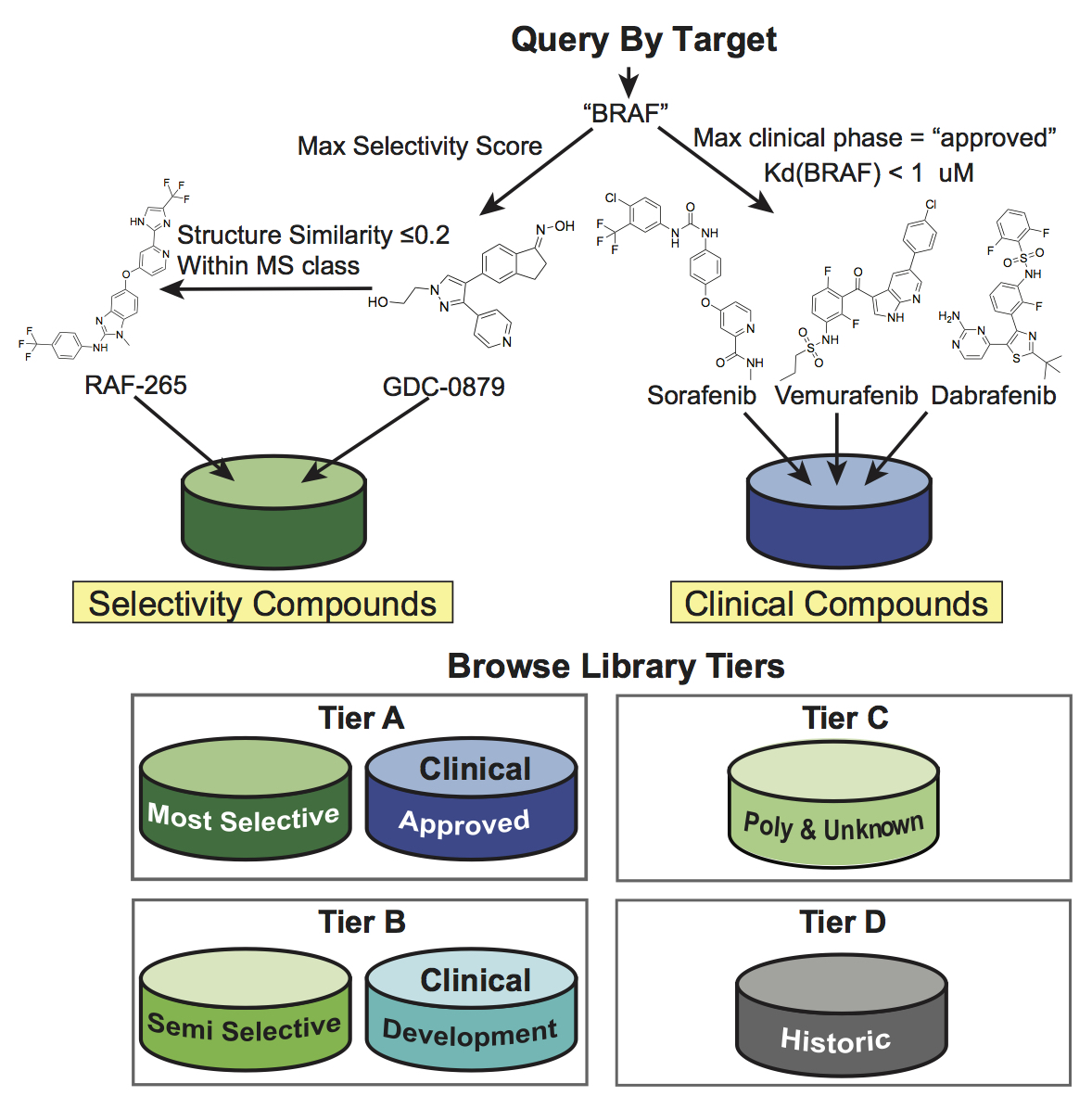
(Upper panel) Illustration of the compound selection procedure for a query target “BRAF” based on selectivity score and clinical phase. In this case, all compounds shown belong to Tier A as they either have MS selectivity or are clinically approved drugs. (Lower panel) Overview of the tiered design of the MoA and LSP-OptimalKinase libraries based on selectivity class and phase of clinical development.
Synopsis
Small molecule libraries are widely used to identify potential therapeutic targets, study biological processes and uncover drug repurposing opportunities. However, screening library optimization—maximizing target coverage while minimizing library size–is challenging: data are incomplete and many compounds exhibit polypharmacology, binding to off-target proteins with unexpected results. We developed a set of data-driven tools to compare compounds and optimize small molecule libraries based on binding selectivity, structural diversity, similarity in cell-based assay activities and stage of clinical development and used these tools to design new libraries that target the kinome and liganded genome. Our approach is shared via open source R code and web-based tools, allowing for further customization of libraries based on user preferences.
Key Findings
- Existing small molecule collections vary greatly on selectivity and target coverage.
- A data-driven approach to library design enhances diversity and library performance.
- Our LSP-OptimalKinase library enhances selectivity and coverage for kinome targets, while our LSP-MoA library optimally targets 1852 genes in the liganded genome.
- Our approach is implemented as R software (www.github.com/sorgerlab/smallmoleculesuite) and a Web-accessible tool (http://www.smallmoleculesuite.org). For futher information on available resources, please see Available data and software section below.
Abstract
Libraries of well-annotated small molecules have many uses in chemical genetics, drug discovery and therapeutic repurposing. Multiple libraries are available, but few data-driven approaches exist to compare them and design new libraries. We describe an approach to scoring and creating libraries based on binding selectivity, target coverage and induced cellular phenotypes as well as chemical structure, stage of clinical development and user preference. The approach, available via the online tool http://www.smallmoleculesuite.org, assembles sets of compounds with the lowest possible off-target overlap. Analysis of six kinase inhibitor libraries using our approach reveals dramatic differences among them and led us to design a new LSP-OptimalKinase library that outperforms existing collections in target coverage and compact size. We also describe a mechanism of action library that optimally covers 1852 targets in the liganded genome. Our tools facilitate creation, analysis and updates of both private and public compound collections.
Available data and software
This paper describes methods to compare libraries and design new ones. In doing so, several key resources were necessary to enable this work. Table S2 gives a mapping table of compound identifiers and target identifiers used by the multiple data sources described in the paper. Table S3 lists the binding assertions per small molecule-target pair, from which target affinity spectra can be constructed. Table S4 lists the members of several target classes (kinases, GPCRs, ion channels, etc.). Table S5 contains two libraries generated in the paper. The LSP-OptimalKinase library is a collection that targets the kinome while the LSP-MoA library targets the liganded genome. Web-based tools are available at https://labsyspharm.shinyapps.io/smallmoleculesuite/>. R scripts are available on Github.
Manuscript Item | Associated Dataset | Synapse ID | HMS LINCS Dataset ID |
---|---|---|---|
Table S2 and Figure 1 | Mapping tables for different compound identifiers and target identifiers. | syn18441416 | |
Table S3 and Figure 4 | Resources to create or customize Target Affinity Spectra. | syn18441417 | 20000 |
Table S4 and Figure 6 | Target class family members. | syn18441419 | |
Table S5 and Figure 6 | Compound lists of LSP-OptimalKinase and LSP-MoA libraries. | syn18441420 |
Funding Sources
This work was supported by NIH grants U54-HL127365 (NM, PKS and NG), U24-DK116204 (NM, PKS), U54-HL127624 (NAC and MM) and by the Novartis Institutes for BioMedical Research (YW, EL and JJ).